U.S. Department of Agriculture: Animal and Plant Health Inspection Service
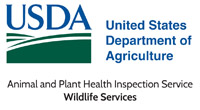
United States Department of Agriculture Wildlife Services: Staff Publications
ORCID IDs
Joshua Hewitt https://orcid.org/0000-0002-0844-7769
Grete Wilson-Henjum https://orcid.org/0000-0002-2284-8745
Derek T. Collins https://orcid.org/0000-0002-3723-2353
Jourdan M. Ringenberg https://orcid.org/0000-0001-9492-3874
Christopher A. Quintanal https://orcid. org/0000-0003-0687-0528
Robert Pleszewski https://orcid.org/0000-0003-1481-0242
Jeffrey C. Chandler https://orcid.org/0000-0002-3318-135X
Thomas J. DeLiberto https://orcid.org/0000-0003-1115-1472
Kim M. Pepin https://orcid.org/0000-0002-9931-8312
Document Type
Article
Date of this Version
2024
Citation
Methods in Ecology and Evolution (2024) 15: 1,677-1,690
doi: 10.1111/2041-210X.14370
Data and code used for this manuscript are available at https://doi.org/10.15482/USDA.ADC/25922608
Abstract
1. Wildlife disease surveillance programs and research studies track infection and identify risk factors for wild populations, humans and agriculture. Often, several types of samples are collected from individuals to provide more complete information about an animal's infection history. Methods that jointly analyse multiple data streams to study disease emergence and drivers of infection via epidemiological process models remain underdeveloped. Joint-analysis methods can more thoroughly analyse all available data, more precisely quantifying epidemic processes, outbreak status, and risks.
2. We contribute a paired data modelling approach that analyses multiple samples from individuals. We use ‘characterization maps’ to link paired data to epidemiological processes through a hierarchical statistical observation model. Our approach can provide both Bayesian and frequentist estimates of epidemiological parameters and state. Our approach can also incorporate test sensitivity and specificity, and we propose model fit diagnostics. We motivate our approach through the need to use paired pathogen and antibody detection tests to estimate parameters and infection trajectories for the widely applicable susceptible, infectious, recovered (SIR) model. We contribute general formulas to link characterization maps to arbitrary process models and datasets and an extended SIR model that better accommodates paired data.
3. We find via simulation that paired data can more efficiently estimate SIR parameters than unpaired data, requiring samples from 5 to 10 times fewer individuals. We use our method to study SARS-CoV- 2 in wild white-tailed deer (Odocoileus virginianus) from three counties in the United States. Estimates for average infectious times corroborate captive animal studies. The estimated average cumulative proportion of infected deer across the three counties is 73%, and the basic reproductive number (R0) is 1.88.
4. Wildlife disease surveillance programs and research studies can use our methods to jointly analyze paired data to estimate epidemiological process parameters and track outbreaks. Paired data analyses can improve precision and accuracy when sampling is limited. Our methods use general statistical theory to let applications extend beyond the SIR model we consider and to more complicated examples of paired data. The methods can also be embedded in larger hierarchical models to provide landscape-scale risk assessment and identify drivers of infection.
Included in
Natural Resources and Conservation Commons, Natural Resources Management and Policy Commons, Other Environmental Sciences Commons, Other Veterinary Medicine Commons, Population Biology Commons, Terrestrial and Aquatic Ecology Commons, Veterinary Infectious Diseases Commons, Veterinary Microbiology and Immunobiology Commons, Veterinary Preventive Medicine, Epidemiology, and Public Health Commons, Zoology Commons
Comments
United States government work