Biological Systems Engineering, Department of
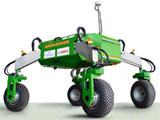
Department of Biological Systems Engineering: Faculty Publications
ORCID IDs
Document Type
Article
Date of this Version
5-2011
Citation
Transactions of the ASABE, Vol. 54:3 (2011), pp. 953-972
Abstract
Understanding the relationship between the spatial distribution of precipitation and crop yields on large scales (i.e., county, state, regional) while accounting for the spatial non‐stationarity can help managers to better evaluate the long‐term trends in agricultural productivity to make better assessments in food security, policy decisions, resource assessments, land and water resources enhancement, and management decisions. A relatively new technique, geographically weighted regression (GWR), has the ability to account for spatial non‐stationarity with space. While its application is growing in other scientific disciplines (i.e., social sciences), the application of this new technique in agricultural settings has not been practiced. The geographic information system (GIS), along with two different statistical techniques [GWR and conventional ordinary least square regression (OLS)], was utilized to analyze the relationships between various precipitation categories and irrigated and rainfed maize and soybean yields for all 93 counties in Nebraska from 1996 to 2008. Precipitation was spatially interpolated in ArcGIS using a spline interpolation technique with zonal statistics. Both measured and GWR‐ and OLS‐predicted yields were correlated to spatially interpolated annual (January 1 to December 31), seasonal (May 1 to September 30), and monthly (May, June, July, August, and September) precipitation for each county. Statewide average annual precipitation in Nebraska from 1996 to 2008 was 564 mm, with a maximum of 762 mm and minimum of 300 mm. Mean precipitation decreased gradually from May to September during the growing season. County average yields followed the same temporal trends as precipitation. When the OLS regression model was used, there was a general trend of linear correlation between observed yield and long‐term average mean annual total precipitation with a varying coefficient of determination (R2). For rainfed crops, 67% of the variability in mean yield was explained by the mean annual precipitation. About 23% and 17% of the variability in mean yield was explained by mean annual precipitation for irrigated maize and soybean, respectively. However, the performance of the GWR technique in predicting the yields from spatially interpolated precipitation for irrigated and rainfed maize and soybean was significantly better than the performance of the OLS model. For both rainfed maize and soybean, 77% to 80% of the variation in yield was explained by the mean annual precipitation alone. For irrigated crops, 42% of the variation in the yield was explained by the mean annual precipitation. For rainfed crops, there was a strong correlation between seasonal precipitation and yield, with R2 values of 0.73 and 0.76 for maize and soybean, respectively. The mean annual total precipitation was a better predictor of rainfed maize yield than rainfed soybean yield. On a statewide average, July precipitation as a predictor had the greatest correlation with yields of both maize and soybean. June, July, and August precipitation had greater impact on maize yield than on soybean under rainfed conditions due to more sensitivity of maize to water stress than soybean. For irrigated yields, July precipitation had more impact on soybean yield than on maize. The performance of the GWR technique was superior to the OLS model in analyzing the relationship between yield and precipitation. The superiority of the GWR technique to OLS is mainly due to its ability to account for the impact of spatial non‐stationarity on the precipitation vs. yield relationships.
Comments
Copyright (c) 2011 American Society of Agricultural and Biological Engineers. Used by permission.