Computer Science and Engineering, Department of
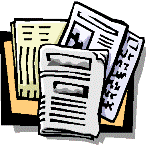
Computer Science, Computer Engineering, and Bioinformatics: Faculty Publications
Document Type
Article
Date of this Version
9-28-2023
Citation
International Journal on Document Analysis and Recognition (IJDAR) https://doi.org/10.1007/s10032-023-00454-7
Abstract
Image downscaling is an essential operation to reduce spatial complexity for various applications and is becoming increasingly important due to the growing number of solutions that rely on memory-intensive approaches, such as applying deep convolutional neural networks to semantic segmentation tasks on large images. Although conventional content-independent image downscaling can efficiently reduce complexity, it is vulnerable to losing perceptual details, which are important to preserve. Alternatively, existing content-aware downscaling severely distorts spatial structure and is not effectively applicable for segmentation tasks involving document images. In this paper, we propose a novel image downscaling approach that combines the strengths of both content-independent and content-aware strategies. The approach limits the sampling space per the content-independent strategy, adaptively relocating such sampled pixel points, and amplifying their intensities based on the local gradient and texture via the content-aware strategy. To demonstrate its effectiveness, we plug our adaptive downscaling method into a deep learning-based document image segmentation pipeline and evaluate the performance improvement. We perform the evaluation on three publicly available historical newspaper digital collections with differences in quality and quantity, comparing our method with one widely used downscaling method, Lanczos. We further demonstrate the robustness of the proposed method by using three different training scenarios: stand-alone, image-pyramid, and augmentation. The results show that training a deep convolutional neural network using images generated by the proposed method outperforms Lanczos, which relies on only content-independent strategies.
Comments
Open access.