UCARE: Undergraduate Creative Activities & Research Experiences
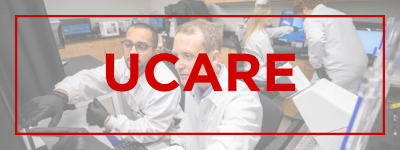
UCARE Research Products
Date of this Version
4-2016
Document Type
Poster
Citation
Marshall, J. E., Richert, M., Mills, M., & Dodd, M. D. (2016). Inferring task based on eye movements: The living classifier. Poster presented at the Annual Undergraduate Research Fair, University of Nebraska – Lincoln, Lincoln, NE).
Abstract
Several studies, including Yarbus (1967), have found that various task instructions for viewing images influence visual behavior. This holds true for both experimenter driven and participant driven tasks. Research has also shown that classifier technology is capable of determining the task that was being performed based on the individual’s eye movements. Typically classifier technology is designed to perform tasks humans are known to be cable of performing. However, little research has been done on the human ability, or lack thereof, to determine task based on eye movements.
Purpose: To determine to what extent humans are able to classify task performed when task was consistent (E1) and when task was switched (E2) based on recorded eye movements in the form of fixations, scanpaths, and dynamic videos.
Task: Each participant completed 60 trials per condition. All participants completed three conditions, fixation, scanpath, and dynamic video. Fixations and scanpaths were displayed for 8 seconds. Dynamic videos were displayed for 4 seconds. Eye movement data was varied between being transposed over the original image or a black background. Participants discriminated between searching, memorization, and rating tasks.
Experiment 1: Task Consistent The eye movement data utilized in the present study was collected from individuals performing only one task (search, memory, or rating) repeatedly.
Experiment 2: Task Switching The eye movement data utilized in the present study was collected from individuals performing the search, memory, and rating tasks intermixed. However, sometimes the same task was repeated at least once.
Comments
Copyright (c) 2016 Jordan Marshall, Mallory Richert, Mark Mills, & Michael D. Dodd.